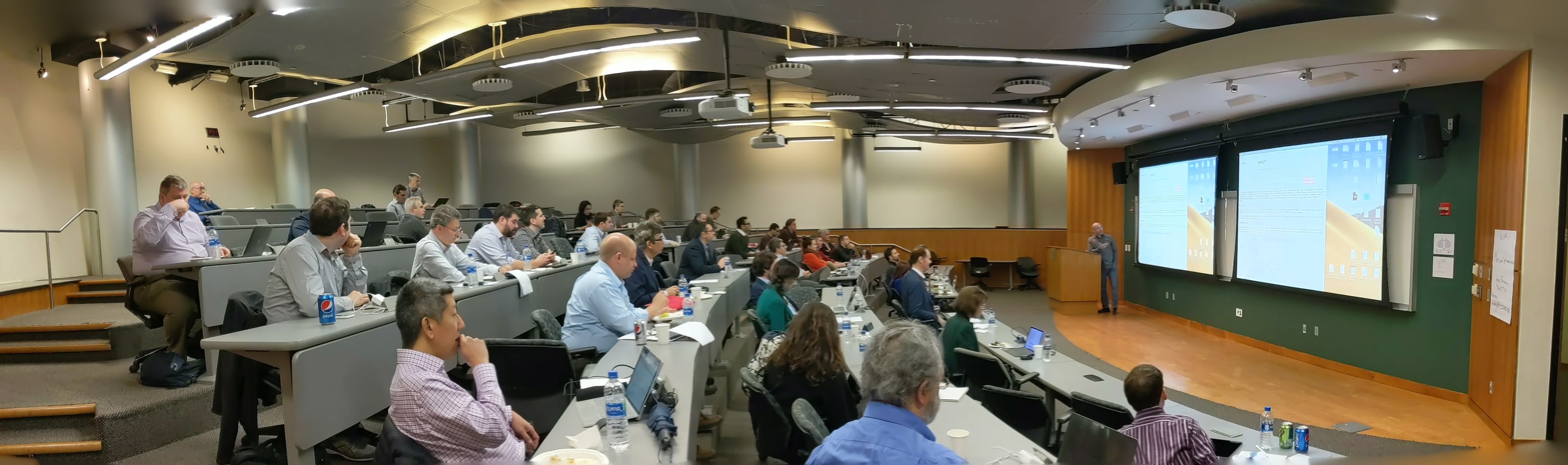
This is the nominal text of my opening remarks for Metricon X, delivered on March 21, 2019. It has been lightly edited for clarity and a few identities have been slightly disguised. The views expressed in this speech do not necessarily reflect those of my present or past employers.
Welcome
I appreciate everybody coming today. It’s a great turnout for a conference that we rather deliberately did not advertise. If you’re here, it’s because you wanted to be here. You’ve self-selected.
The theme of the conference is “plus ça change…,” the second half of which is “plus c’est la même chose.” Colloquially: “the more things change, the more they stay the same.” So what we’re really here to talk about are the constants and the change. But because I suspect that we will have ample time to reheat some of the old chestnuts (the constants), I’d like to offer a few remarks on the changes — that is, notable happenings in the world of security metrics over the last 12 years.
Data-driven security took root
One of the most gratifying things to emerge in security over the last 10-plus years is the increased fluency and comfort people have with real security data. This is not completely new. Bill Cheswick’s work at Bell Labs in the late 1990s on network mapping, for example, helped create a company (Lumeta) that specialized in analyzing networks, and developed a specialty in analytics for use in M&A situations. Jim Cowie, formerly CTO of Renesys, as another example, was doing large-scale analytics on BGP routes at the turn of the millennium. The last dozen years has brought many more examples, notably:
- The Verizon Data Breach Investigations Report (DBIR), which fused together law-enforcement data and private sources to paint a data-rich picture of what data breaches look like, are caused by, and cost. The DBIR, and publications such as Larry Ponemon’s eponymous studies on breach costs, helped popularize a metric known as “cost per record.” As a result, we now have relatively well-accepted currency for calculating potential and actual consumer information exposures.
- Observables and ratings. Spurred on, in part, by the challenges of the the questionnaire-based approach to evaluating vendor security, vendors such as BitSight and Security Scorecard have focused on inferring the security of companies based on what they can empirically observe. If your MX and DNS records are messed up, or if spam is coming from IP address space you control, or if externally-facing systems appear to be compromised, then the rest of your security program probably isn’t any good either. Ratings are derived from how spotless one’s external presence is. Data about your supply base, for example, can help you make a decision about when need to dispatch the goon squad to interrogate a high-risk vendor.
- The increased use of statistical and data science tools to analyze large security data sets. These include Python (eg PANDAS and NumPy), and the R ecosystem, the HadleyVerse and so on. There are a healthy number of “R-heads” in the security metrics community, such as Jay Jacobs, Bob Rudis and many others. I count myself among them. Although many of the studies are custom-made, the prevailing attitude is to practice reproducible science using a tool-driven analysis and workflow. Find interesting problems and data sets. Explore them. Publish findings. Repeat!
And also, somewhere along the line, data science became a Thing. Some of us used to call it “statistics.” Speaking of which…
“AI” has come to security, with uneven results
“AI” has come to security, with uneven results. I say “AI” in quotes because what we call AI in the popular press is not about endowing computing machines with cognition. I must tell you, every time I see that Microsoft commercial with the rapper Common extolling the virtues of “AI,” I feel like Marvin Minsky spins another turn in his grave, and that Douglas Hofstadter rips up and crumples one of his piano compositions and weeps.
Once you get beyond the commercials, “AI” is primarily about creating models to make better predictions, using a bag of tricks that includes supervised and unsupervised learning, neural networks, bayesian strategies, Markov networks, bootstrapping, anomaly detection, and a whole set of other buzzwords that many of our attendees have better first-hand experience with than I.
In security, many of these “AI” techniques are being put to use to help solve some very real operational security problems, for example, making a security operations team more efficient. Consider an enterprise-class SOC with dozens of analysts. The sensor grid will ingest daily log volumes in the tens of millions, extract tens of thousands of potentially suspicious activities, and then reduce these down to dozens of cases to put in front of human analysts. As a rule of thumb, it’s about roughly one million pieces of straw in every haystack, for each needle found in it.
Financial services and national agencies are two types of organizations that have the threat volume, funding and organizational capability to fund vendor and internal efforts in this space. They have big haystacks and lots of needles to find. A large focus of research and vendor efforts is in increasing the signal-to-noise ratio. From a measurement perspective, this means using “AI” to correctly classify genuine intrusions (true positives) and non-intrusions (true negatives), and reduce the false-positive and false-negative rate.
But results have been “uneven” because it’s a tough problem space. Many vendors will tell you that they’ve got bulletproof, universal techniques that solve all sorts of superficially related problems. For example, network intrusion detection and insurance fraud are both anomaly detection problems, right? I’ve heard a vendor say, “well, our AI/neural net/ML engine solves both of these problems.” Actually, they are in different domains and have very different characteristics in terms of variety of data sources, completeness, and outlier detection strategies. There is no one size fits all. I’m inherently suspicious of generalizable AI in security. But every time I see a well-bounded, domain specific strategy, I’m happy.
In addition, there is lots of low-hanging fruit that can be harvested by simply fusing data together at the presentation level to make investigations more efficient. SOC labor optimization is more like an operations research problem than an “AI” problem. With respect to making SOCs more efficient, there’s plenty of room for experimentation at both ends of the funnel, by attacking the top and middle of the funnel to present the truest and most accurate incidents; and then, improving the efficiency of the investigations of the cases that fall through to the bottom of the funnel.
Success disasters are great teachers
Dr Dan Geer first introduced me to the concept of a “success disaster”; something that goes so well that it creates painful side-effects. Here in New York, you could argue that the cronut craze that began in 2013 was a success disaster for the Dominique Ansell Bakery. Sure, there were lines around of the block, but it led to a black market in resellable cronuts, counterfeit cronuts, quotas for cronuts, and I am sure, staff burnout and ingredient shortages. It was also a disaster for ordinary customers. If, for you, the Ansell Bakery had been a lovely place to have your morning French roast while leisurely enjoying a croissant, reading Le Monde and chain-smoking Galois cigarettes, it is no longer. That dream was trampled by all of the marauding tourists.
In security metrics, it’s been gratifying to see a lot more focus on data, analytics and metrics. And many of the metrics I’ve been seeing are much better than the stuff that drove me batty when I wrote my book twelve years ago. You know, stuff like turning highs, mediums, lows into cardinal numbers like 5, 3, and 1, or (worse) 9, 3 and 1, and then doing math on them and claiming the results are “quanty.” Or creating an “index” that uses mystery math to jam a bunch of semi-related indicators into a score that can’t be easily explained, on the theory that because the Dow Jones Industrial average is an index, and we all know that a higher means we’re richer, then our security metric needs to be an index too. These are mistakes anybody can make, and usually do when they start off.
Many organizations have matured their thinking and have gotten religion about measuring things. At a bank I’m familiar with, for example, the GRC team produces a 100-page monthly pack of metrics that cover all areas of technology risk. Many of the metrics count things things that risk or control owners consider important, typically trailing indicators, often with breakdowns by organizational units, and almost always with commentary and correct attribution about sources. The 1,000 or so metrics in this pack are assiduously collected every month and assembled into a polished report. This is wonderful. It is a success. It is also a disaster, because the quantity of data is challenging to assimilate. It is challenging to see the forest for the trees.
Here’s another success disaster: vulnerability management. Everybody in the audience knows what a vulnerability scan is, and what it does. It finds weaknesses and exposures in technology assets, typically on endpoints such as servers and desktops. The tools have gotten very good and produce few false positives. What’s more, there’s a general consensus on an industry-wide rating scheme for measuring severity: the Common Vulnerability Scoring System (CVSS). The market is mature, with well-established vendors such as Qualys, Rapid7 and Tenable.
What’s not to like about vulnerability scanners? They have a consistent measurement system, are accurate and pervasive. If the scanner says something is bad, it must be right? We should fix all “critical” vulnerabilities right away, shouldn’t we? Sounds great. But the problem is that there are too many darned vulnerabilities: millions in the typical large enterprise. What do you fix first? This is very much a success disaster.
These kinds of problems are excellent teachers, because they force you to think differently about the problems. In the vulnerability management space, for example, one must begin with the concession that not all vulnerabilities are cost-effective to fix. Some matter more than others. How important is the asset they are on? And is the vulnerability weaponized? Are attackers actively exploiting the vulnerability in the wild? Both of these are tedious and error-prone processes to do as one-offs, but can be attacked with a bit of engineering. So now you have vendors such as Kenna (founded by one of securitymetrics.org’s early members, Ed Bellis), applying logic over-the-top of the scanners you’re already using. Maybe you don’t need to fix 1 million vulnerabilities. Maybe this week, the only thing you worry about is the one-half of one percent of the vulns, or 5,000 patches relating to a single CVE that other companies are seeing abused by scripted attacks. That is a nice win, even better than the proverbial 80/20.
For coping with success disasters in areas such as risk and control issues, I tend to worry less about the overall numbers of issues, and focus more on the pockets of risk “debt” that aren’t being paid down. Suppose you’ve got 10,000 risk issues and control breaks on the books, across the whole company. That sounds like a lot, but only 250 of them are in your highest-severity bracket. What’s the best way to figure out which ones to attack?
There are many ways to look at the data — for example, finding who has the largest number of high-severity issues, or those with the largest number of longest-aged ones. Mean-time-to-close is another. Personally, I like “velocity” as the right way to look at the problem. Who’s paying down debt fastest, and who’s letting it sit?
I stole a metric from the warehousing industry called “turnover,” which is defined as the number of SKUs flowing through a warehouse, divided into the average inventory. For example, Apple’s inventory turnover in 2017 was 60, meaning it sold through everything in its warehouses every 6 days.
When adapted for issue turnover, we define it as the number of closed issues divided into the average inventory. You don’t get credit for issues you postpone or renew. So for example, if you start with 100 issues on Feb 1, and end with 120 on Feb 28th, that’s bad, right? But what if you closed 65, and added 85? That’s pretty good, because you closed half of your issues during the month. Your issue turnover is 0.5, or when expressed as an annualized figure means your inventory would turn over 6 times per year. That’s actually quite outstanding. Now imagine computing issue turnover by organizational unit and severity of issue. You’d see the high and low performers right away.
This issue turnover metric works well because it is easy to understand and rewards the behaviors we want to see: paydown of issue debt. This is another example of how a success disaster causes us to evolve our thinking, and allows us to prioritize better.
Controls instrumentation offers terrific bang for the buck
When I joined a large investment bank as the MD for technology risk measurement and analytics, I was excited that I’d be able to put some of my ideas about security metrics into practice. I’d done a fair bit of metrics work on a smaller scale in prior roles, but the bank had both the commitment and the resources to do it properly. But what I found out quite quickly after coming in was that the primary use of “metrics” was in demonstrating controls conformance, chiefly for Sarbanes-Oxley and assurance régimes such as SSAE-18. Our biggest customer wasn’t the security organization — it was our external auditors. They needed our data to be able to show quantitatively that the key controls were working. Our second biggest customer was the finance organization, because they ran SOX, although they were less interested in the data than the results.
The “sweet spot” for the continuous controls monitoring program was identity and authorization, which lies at the heart of technology risk management. “No privilege without identity. Approve all privileges. Remove them in a timely manner when roles change or someone leaves the firm.” These were well-instrumented operational processes with well-defined systems to tap for the data. Because we calculated control effectiveness at a very granular level, we could state with confidence whether a particular control was effective or not. We had the data to prove it. No arguments.
A key insight the team had was to being applying a similar approach to a large annual process that many of you are intimately familiar with, the Risk and Control Self-Assessment (RCSA, or as my contact from the Fed calls it, the “ricksa”). If you’ve had the pleasure of doing one, it’s usually an annual exercise that touches the entire enterprise. Both business-managed and control-function-managed controls are included. Everybody does it a little differently, but the basic steps are similar: (1) define “assessment units” that will perform the risk and control assessments; (2) set up the ratings scales for assessing inherent and residual risk; (3) have each assessment unit assess their inherent risk; (4) have each control owner assess the controls that help reduce these risks; (5) synthesize the results, calibrate them, determine residual risk and roll everything up.
All of this sounds nice in theory, but the defects in practice are known.
- Because so many people are involved, RCSAs can’t be done regularly; at most, most organizations will do them once a year.
- Because the ratings are subjective, a lot of time is spent “calibrating” and “challenging” to try to ensure that nobody lied particularly egregiously. And,
- Because of time constraints and the lack of detailed empirical facts about the control environment, assessors must evaluate in a very coarse-grained way, perhaps, at a sub-line of business level at best. What this means is that a significant risk or control weakness affecting a particular asset is steamrollered over by the tyranny of averages.
In short, these RCSA exercises aren’t timely, objective or precise. So what good are they? Based on comments from practitioners, not much good at all. And the regulators know it, which is why they are quite openly fishing for alternative approaches.
What we found was that applying the continuous controls monitoring strategy to RCSA offered a terrific bang for the buck. The key was to do it in a commercial way. For example, consider Dorian’s wonderful Unified Compliance Framework, which offers a consistent and universal taxonomy of controls that can be mapped to every technology or cyber framework, regulation or statute. If you pick just three of these mandates, for example ISO 27000, the EU’s General Data Protection Regulation (GDPR) and the NIST Cyber-Security Framework, UCF will tell you that you need something like 600 controls, with another 300–400 implied. You would never want to automate the measurement of that number of controls. That would not be commercial, and you’d never be done.
Instead, why not pick the 50 technology controls that we know from experience offer the biggest risk reduction potential, and instrument just those? We developed a playbook, which went more-or-less like this: “hey subject matter experts, we think change management, software lifecycle, data quality, tech ops, asset management, intrusion detection etc etc are the most important risk areas. How would you define ‘success’ in these areas? What metrics can we agree on that describe success? Who owns the data?” And then: defining a project plan for sourcing, loading, transforming and refining the data, in waves, so that we can compute the metrics we agreed constitute success. As a sweetener, we bribed the data owners with free labor to get their data into the computing plant.
There are some caveats:
- The data is never complete, but that’s ok, because it’s good enough to be indicative… and certainly better than “1-5 scales” that are based mostly on opinions leavened with a few facts.
- The early results are always ugly, but that’s ok, because un-instrumented controls are always ugly the first time one sees the data. But nobody ought to get fired if the data’s all new and the control implementers haven’t been given time to fully adopt or get their performance in shape.
- And it takes time, but that’s ok, especially if one sequences the plan to deliver quick wins first
In short, having a rigorous plan to delivery incremental value of a small number of representative metrics makes assessment processes more timely, precise and objective. It’s important to keep the exercise limited to key controls that you can tangibly measure. And it is critical to keep reminding everybody about all of the cost and complexity that’s being removed — typically, millions of dollars of labor that is largely guesswork.
Audience is everything
People want data for different reasons. And people consume data differently. What might seem good to you might be Greek to someone else. As a rule, I believe that when we build exhibits and reports, we tend to condescend to the reader. We assume that if we don’t lard exhibits with lots of reds, yellows, and greens, the person who is reading it won’t get it. Or we use simple pie and bar charts that waste space and are not data-dense. I ranted about this in my book a long time ago, but it’s still true. I rarely see information graphics related to security metrics that are more complicated than one-dimensional, for example, categorical data displayed as a bar chart. This is understandable in many ways, because most information graphs used in high-volume reports don’t need to do too much. They’re not there because they provide a lot of diagnostic power. They are meant to just get a simple message out. But is the message even right? If you don’t know who your audience is and what they want, it can’t possibly be — and so you are forced to keep it simple. If you knew your audience better, you could take them along much further, with more relevant and powerful metrics.
When I look at published metrics and exhibits, I ask five questions that have a simple mnemonic: A-B-C-D-E.
- A is for Audience. Do we know who we’re putting our metrics in front of? Do we know what they want?
- B is for Behaviors. If you’re looking at a chart of exhibit, what behaviors do I want the audience to change based on the inferences or conclusions in the data?
- C: can I Concisely and clearly communicate, in the simplest way possible, the data I that the audience will need to make…
- D: …the Decisions based on the data I put in front of them?
- E: Lastly, does my data include commentary with an Editorial voice that showcases my expertise and provides context to guide the audience to the decision?
Because Audience is everything, you have to start there. That’s a key lesson I’ve learned personally over the last dozen years.
Outside of the security field, I two relatively new disciplines have emerged as Things that people specialize in that relate to the question of Audience. The first is data visualization as a discrete field of study, and a sub-field related to information dashboard design. For data visualization (or “data vis”), toolsets such as Tableau, D3 and GGplot have turned visualization into a rich grammar that can be programmed, layered and reused. And websites like Information Is Beautiful and Flowing Data celebrate novel ways of mashing up and showcasing data. Stephen Few has been doing pathbreaking work on dashboard design — I can’t recommend his work highly enough, because of the rigor with which he approaches make-overs of the sorts of dashboards that we are all showing our bosses. As security and risk professionals, we all benefit from the increasing formalism of the field of data visualization, and from efforts to promote more “visualization literacy.”
Data journalism is the second Thing I’ve been following that benefits our field, and it too relates to Audience. Made mainstream by Nate Silver’s FiveThirtyEight election prediction work, nearly every premier news publication has invested in what is now called data journalism. Data journalists are either quants like Nate who happen to write persuasively, or data-curious journalists that got their Nerd on and developed a niche. The essence of data journalism is telling stories with data. Notable publications that are doing this really well include the New York Times, which has been doing some extraordinary data journalism over the last ten years; the Economist, which has always had excellent, honest, sound data graphics but has recently gone much deeper into analytics; and of course, the now-ESPN-owned fivethirtyeight.com. And academics such as Alberto Cairo are also doing incredible work in this space.
A few years ago I made a highly speculative hire — I hired the head of the data journalism team from a major business publication. The theory was, we’ve got lots of data, but we’re doing a crap job telling the story. Let’s see if we can bring in someone with a hybrid skillset. She writes well, and fast — is used to writing on deadline. As a reporter, she’s got a nose for the headline. And she’s got data chops. Maybe not like a full-on data scientist would, but hey, give it time. It turned out she was exactly what we needed. It was a true win-win… the bank got a massive upgrade in clarity and impact. And my new team member was happy as a clam because by making the jump into financial services, we were also able to raise her compensation by a very healthy amount.
The point I’m trying to make here is that the skills that made our data journalist such a valuable member of the team was, more-or-less, ABCDE. In short: knowing your audience, what they want, and what you want out of them. And then, constructing the simplest and most efficient narrative that encourages inquiry, while also making setting the stage for decisions that shape behavior.
This talk was meant as a retrospective, so I could have talked about any number of things. I mentioned these five trends…
- data-driven security
- “AI” in security
- success disasters as teachers
- controls instrumentation
- audience focus
…because they represented topics that I’ve learned a lot about, and that have benefited the industry. Thanks for listening to this rather old-school speech — no slides — and I look forward to seeing what Metricon XX will bring.